# Key Pitfalls to Avoid in Data Engineering for Success
Written on
Chapter 1: Understanding Data Engineering Challenges
Data engineering plays a vital role in equipping organizations and teams like data scientists with essential data. Professionals in this field create data pipelines and platforms, which are currently in high demand. However, there are several critical errors that data engineers should steer clear of to enhance their effectiveness.
"Ensuring data quality is paramount; poor data can lead to misguided insights."
Section 1.1: Prioritizing Data Quality
Maintaining high data quality is essential for the success of any data engineering initiative, influencing subsequent processes like data science, machine learning, and business intelligence. Without accurate and reliable data, the insights drawn can be erroneous or deceptive. Therefore, focusing on data quality must be a fundamental aspect of any data engineering project. This can be achieved by implementing thorough data validation, profiling, cleansing, and monitoring practices. For additional insights, consider this resource:
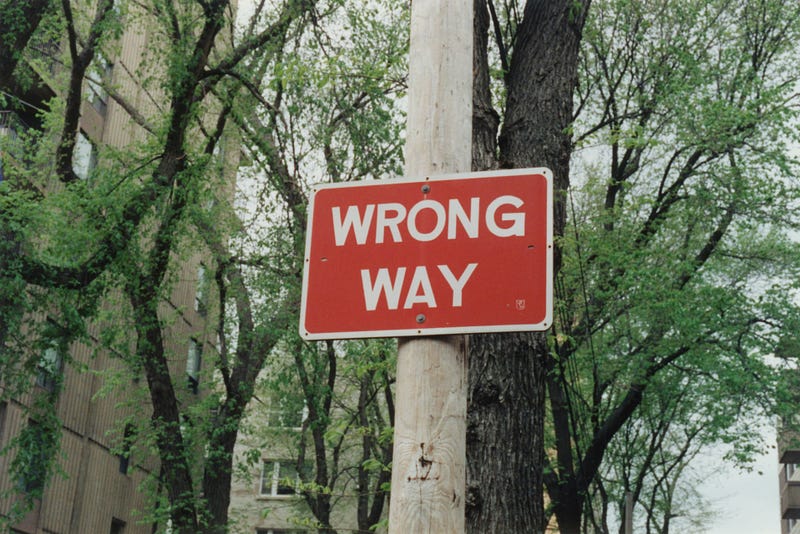
Section 1.2: Safeguarding Data Privacy and Security
Data privacy and security are crucial components of any data initiative. A breach can result in significant financial and reputational repercussions for an organization. Compliance with regulations such as GDPR and the California Consumer Privacy Act is essential, as non-compliance can lead to hefty penalties. Therefore, data engineers must adopt secure methods for data transmission, storage, and processing. This includes using encryption, implementing access controls, and employing monitoring tools to detect and respond to potential threats.
The first video discusses the potential downsides of a data engineering career, highlighting common pitfalls and strategies for avoiding them.
Section 1.3: Emphasizing Data Governance
Data governance is vital for managing data within an organization, encompassing ownership, usage, and policies. Ignoring data governance can lead to inconsistencies and poor data quality. Implementing effective governance procedures is crucial to ensure that data remains accurate, consistent, and compliant with relevant standards. In the context of data lakehouses, strong governance is necessary to prevent them from becoming data swamps, ensuring that the right data reaches the appropriate stakeholders.
Section 1.4: Planning for Scalability and Modern Architecture
As data volumes and use cases expand, designing a data platform with scalability in mind becomes increasingly important. Solutions like data lakehouses can offer scalable, cost-effective options that adapt to diverse use cases. Such modern architectures not only support data governance but also empower employees to make informed, data-driven decisions. The concept of a data mesh can be effectively integrated with the data lakehouse approach to enhance scalability.
Summary: Key Takeaways for Data Engineers
In summary, avoiding these common pitfalls in data engineering can greatly enhance project efficiency and reliability. By prioritizing data quality, ensuring privacy and security, implementing strong governance practices, and planning for scalability, data engineers can contribute to successful and impactful data projects.
The second video explores the do's and don'ts of Airflow and analytics engineering, providing valuable insights for effective practices in the field.
Sources and Further Readings
[1] Datenschutz.org, BDSG & DSGVO: Welches Bußgeld sieht der Bußgeldkatalog zum Datenschutz vor? (2023) [2] Baker Hostetler, The California Consumer Privacy Act: Frequently Asked Questions (2023)