Navigating the Complexities of P-Hacking in Research
Written on
Chapter 1: Understanding P-Hacking
P-hacking is a phenomenon where researchers repeatedly analyze data until they find a statistically significant result, which they then present as valid. This practice can lead to misleading conclusions and undermines the integrity of scientific research.
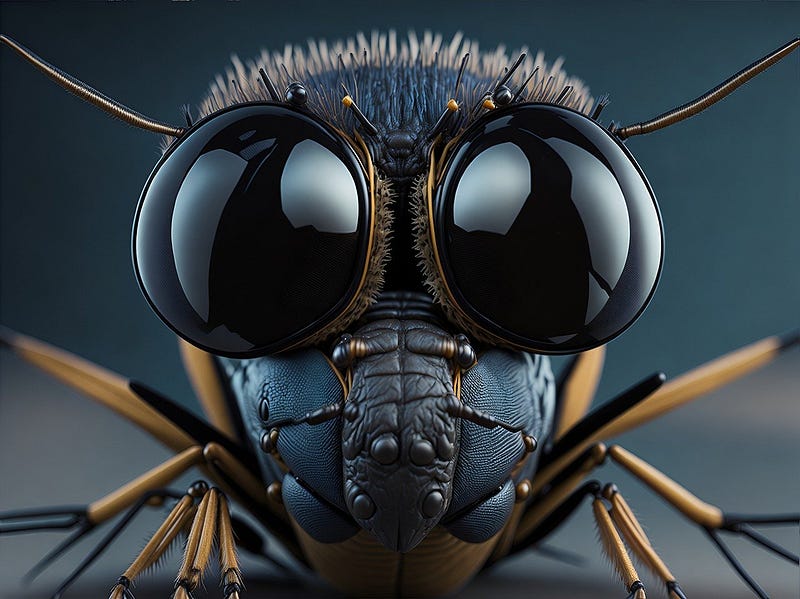
Hand a random dataset to an analyst, and they can craft a narrative as persuasive as one derived from genuine data. The term "p-hacking" describes the act of conducting multiple tests on the same dataset until a statistically significant outcome is found. This often involves manipulating various elements within the analysis to achieve desired results.
A "p-value" plays a crucial role in this context; it indicates the likelihood that a given result is due to chance rather than a true effect. While hypothesis testing provides a structured approach to validating results, we engage in a similar process in our everyday lives without conscious awareness. Essentially, problem-solving can be viewed as a series of hypothesis tests.
Researchers commonly rely on p-values to decide if their findings are noteworthy. When an initial analysis fails to meet the required threshold, p-hacking can occur through various methods, such as:
- Altering the variable under examination.
- Redefining the variables to fit a more favorable outcome.
- Modifying the population sample to find a subgroup that yields significant results.
- Conducting statistical tests across multiple subgroups, increasing the likelihood of false positives.
- Lowering the significance threshold and reporting findings as directional, despite not meeting the conventional 5% standard.
P-hacking often arises from understandable motivations. External information may suggest a certain result should be evident, and the large sample sizes available today make it relatively easy to find statistically significant outcomes. Hand me a dataset of 2,000 respondents, and I can certainly uncover significant results that would impress any marketing team.
Effective analysis should not involve arbitrary adjustments to data. Unfortunately, many researchers fall into the trap of tinkering until they find a working solution, neglecting to document the numerous unproductive routes they take. Statistically, one would expect to obtain a significant result by chance about 5% of the time.
To avoid the pitfalls of p-hacking, researchers should be vigilant about their methodologies and strive to design studies rigorously, minimizing the temptation to manipulate data. Misusing statistical analysis can lead to erroneous conclusions, such as falsely rejecting the null hypothesis.
For example, consider the results of several vaccines:
- Vaccine A yields a p-value of 0.2
- Vaccine B: 0.058
- Vaccine C: 0.4
- Vaccine D: 0.02
- Vaccine E: 0.07
A simplistic interpretation might suggest that Vaccine D significantly reduces recovery time. However, making such a claim could be a case of p-hacking, potentially resulting in a false positive. This is particularly critical in an era where AI tools are constantly evolving.
The first video, "P-Hacking: Crash Course Statistics #30," explores the concept of p-hacking in detail, discussing its implications in research and the importance of statistical rigor.
Chapter 2: Avoiding P-Hacking
Understanding the perils of p-hacking is crucial for maintaining the integrity of research.
The second video, "P-hacking: What it is and how to avoid it!" provides practical strategies for researchers to avoid engaging in p-hacking and emphasizes the importance of sound statistical practices.